There has been a lot of change in financial crime over the past 10 years, but one thing that’s remained constant is the problem of too many false positives. The nature of the beast is that there will always be false positives. Separating money laundering from normal behaviour is a difficult task.
If your transaction monitoring is creating too many unnecessary alerts to process, then you are wasting your team's time. The impact is that your analysts may not assess the significant alerts properly because they have too much to do in too little time. By reducing the number of alerts, you are giving analysts more time to properly analyse the alerts that might be true positives that need reporting.
It may seem obvious, but to identify false positives you must look for them. I’ve seen too many cases where teams continually close down masses of alerts without thinking about whether they should be getting those alerts in the first place.
This could be the job of a team member assigned to look at the big picture of the output of rules, but it can also come from each analyst raising the issue of unnecessary alerts, instead of putting their head down and processing them. They are in the best position to know what is happening, so empower them to raise concerns whenever they have them.
What is a false positive?
A false positive could be defined as:
“An alert that is incorrectly raised because of an imperfect rule”
The rule might be imperfect because of what it is attempting to find, the way it is written, the thresholds it is using or the quality of the data.
The converse is a true positive, where the rule picks up a crime that needs reporting.
In black and white terms, we want our rules to detect true positives and not false positives. However that is too black and white, there are a lot more nuances.
Identifying false positives
Rather than thinking in black and white terms of true and false positives, I like to grade the alerts on a scale from true positive to false positive. Instead of having two outcomes let’s put the alerts into five categories on a simple linear scale of 1 to 5.
- Category 1 are the cases that are definitely a crime (black and white case of true positive).
- Category 5 are alerts that we never want to see again (black and white case of false positive).
- Category 2 is where something looks somewhat suspicious, but it doesn’t meet our threshold of reporting. Given this alert we might put the customer on watch or wait until a similar alert is raised for the customer and perform a more thorough investigation then. In the black and white view, this is a false positive. But I would argue that it is a good outcome that we want to see.
- Category 4 is something that might be an issue, but on further analysis turns out not to be, as a short investigation reveals that it all appears above board. This is still a false positive.
- In the middle there (Category 3) is always the “not sure” case.
If we are counting false positives, which of these cases should we count? If someone claims to have a 90% false positive rate, does that mean the 90% are in categories 2-5, or 3-5 or 4-5? To my mind, categories 4 and 5 are false positives that something should be done about. I wouldn’t include category 2 and I would sit on the fence about category 3.
If you haven’t categorised your alerts, I suggest that you retrospectively categorise them. You don’t have to do it for all your alerts. Pick the rule that you think is your biggest problem and categorise the alerts for that rule for, say, the last month.
Now that you have categorised the alerts, you are in a good place to start doing something about all those false positives. This is where a lot of people come unstuck – they don’t actually do anything about them. Make sure you put aside the time to do a proper job.
Reducing false positives
Every case is unique, so each case needs to be treated on its own. I don’t have all the answers, but there are some common places to start.
Treat each rule separately and give a thought to the outcome that you want. For example, if you have a rule looking for a high consequence crime, such as child sexual exploitation, you might decide that you are prepared to accept a higher than usual number of false positives to ensure that you don’t miss anything.
Remember that the outcome you are looking for isn’t to match the number of alerts to the size of your team. The outcome is to remove the alerts that are a waste of time.
Some rules may jump out as the obvious place to start. For example, if a rule resulted in lots of alerts that were all in the bad category 5, or maybe most alerts were in categories 4 and 5.
Pick one rule and work on that. It doesn’t matter which rule you choose, but ideally either one that has many false positives or one that you think is easy to fix. The key is to pick one and only one. Make changes until you have finished with that rule. Then move onto the next rule. Don’t get caught up trying to make too many changes at once. Keep it simple, do one thing well before you attempt the next.
Is the rule actually doing what it is intended to do?
The first thing I check is whether the rule is actually doing what it’s intended to do.
This kind of situation is relatively common. I’ve seen plenty of cases where the rule, the documentation and the risk assessment don’t line up. The analysts have no idea what the rule is supposed to look for and continue processing it assuming it has been set up correctly.
The other angle on this is whether the rule is written or coded correctly. It’s very easy to make a mistake when setting up a rule. So always make sure you test changes to a rule before you make it live.
Are the thresholds suitable?
Let’s now assume the rules are all well written and look at some other situations.
A very common situation is a threshold that is too low.
You should compare the amount of the alerted transaction with the category. If everything in category 5 has a low amount, that would suggest that the threshold is too low. You should be able to compare all the amounts with the category they are in and work out what threshold is safe to move up to.
If you don’t feel brave enough to raise the threshold straight away, split the rule in two, one above the new threshold and the other between the old and new thresholds. That way you don’t miss anything, but you get a clearer view of the value of the threshold. If the rule between the old and new threshold always turns up junk, it will give you more confidence to move up to the new threshold.
Segregate your customers
Does that threshold apply equally to all customers. To businesses and people, to high wealth and low wealth customers, to the young and old? A high amount might be quite normal for businesses or high wealth customers, but not for the youth or unwaged. By analysing the customer types in each of the alert categories, you may decide to vary the thresholds.
You can create other segmentation as well. For example, I have a client who manages several funds. They have separate rules for each fund. The rules are essentially the same, but the thresholds are different.
Data quality is vital for good results
Poor data is a big issue for lots of people. To get good results you need good data.
If you know that your data is incomplete or unreliable you can possibly handle it, but if you don’t you are running blind.
Review, modify, test, action
Failure to do something about false positives is often the biggest problem. Don’t fall into that trap. When you find a problem, raise it and work out a plan to deal with it. Then enact the plan.
- Review your alerts for a rule and plan what you want to do about it.
- Modify the rule to meet your hypothesis of what a better rule looks like.
- Test the rule to see if reality matches your theory.
- If the results of your testing are good, then take action and make your changes live. If not, go back to the review stage.
Even if your change is good, this isn’t a once round the cycle. Keep reviewing your alerts, especially after you action the new version, but also continually as your business, criminal behaviour and the world at large changes around you.
To summarise, reducing false positives in your AML programme requires a strategic approach. Firstly, it's crucial to understand what a false positive is—an alert incorrectly raised due to an imperfect rule. To address this, it’s important to establish a clear process for identifying and tackling false positives, don’t just leave this to chance. Taking a systematic approach, focus on one rule at a time, and apply the approach review, modify, test, and action.
The evidence is often clear, but it's essential to actively address the issue instead of simply enduring it. Empower your team to raise concerns, categorise alerts effectively, and allocate dedicated time to reduce false positives. Remember, each case is unique, so tailor your approach, prioritise effective rules, and regularly review and adjust as needed.
Get in touch with us!
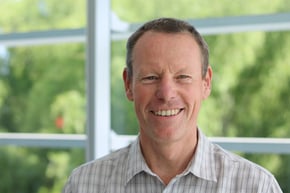