Last week I had the pleasure of being part of UK Finance’s inaugural Fraud Technical Briefing, where Jade ThirdEye hosted a panel discussion about changing monitoring tactics to identify victims of financial crime, with valuable insights from my fellow panellists Diane Briggs De-Beere from Yorkshire Building Society and Mark Courtney from CIFAS.
There were also enlightening discussions about tackling the problem of money mules in the context of the changing liability (Daniel Holmes, Feedzai and Gareth Pindred, Virgin Money) and Contact Centre based attacks (Tim Burton, Smartnumbers, Dan Standish, Santander and Matt Smallman, author of Unlock Your Call Centre).
There were a number of themes running throughout the day but for this blog, I’d like to focus on the value of using non-monetary data within your customer, account and transaction monitoring.
Looking at examples to help you identify patterns can be done manually or with the assistance of machine learning or AI, but the principle is the same. What lessons are there to learn? What opportunities are there for you to flag a high-risk customer, account or transaction? Ideally early enough to prevent any loss, but certainly to minimise financial gain by the criminal. After all, disrupting the flow of criminal money and denying the fraudsters their reward is what drives us as financial crime professionals!
In the example of money mules, speed is key. Money will flow in and out of accounts in minutes rather than hours or days, so think about how can you identify which inbound payments present the highest risk. Under the forthcoming APP liability shift, monitoring incoming payments takes on a new focus.
Think about what other behaviours might be evident in each scenario, and what data do you have to add to your monitoring? In the mule example, it could be behavioural anomalies such as repeated balance checking through calling an IVR or checking online, at an ATM or in branch. It could be device analytics or geolocation data which, when added to transactional information such as a payment from a previously unknown source, elevates the risk and gives the opportunity for intervention before the funds are moved on.
Depending on the size and nature of your organisation, you may have teams of people specialising in different types of financial crime, you may have one person covering AML, Fraud, Sanctions, Compliance and Conduct Risk or most likely somewhere in between. Whatever your crimefighting resource profile, it’s well worth the effort to take some time out and use their experience. Get them to share the cases they’ve investigated. Share case studies with your network and learn from each other, and then take those examples and look at the data available to augment your monitoring.
If you’d like to chat with the team here at Jade ThirdEye about how we can help you to get the best from your data to fight financial crime, please use the form below to get in touch.
Get in touch with us!
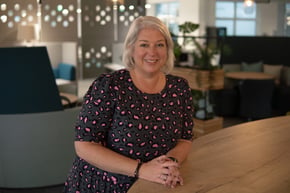